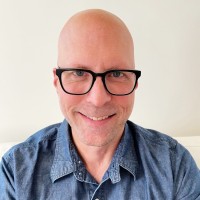
This blog highlights the key points we discussed in our recently concluded webinar on O-RAN and its business opportunity. The proliferation of cloud-native technologies and the adoption of open source in 5G networks are driven by the need for scalability, flexibility, service orchestration, cost efficiency, collaboration, and interoperability. These trends help address the challenges posed by the scale and complexity of 5G deployments and foster innovation. The cloud native approach allows for the efficient allocation of computing resources, enabling dynamic scaling and on-demand provisioning to meet varying network demands.
O-RAN the Final Frontier
O-RAN is the "final frontier" for Network Operators' open, interoperable, virtualized, and disaggregated HW+SW solutions. By embracing O-RAN, network operators can break free from the traditional closed and proprietary network architectures. They gain the ability to select and integrate components from different vendors, promote innovation, reduce costs, enhance security, and ensure long-term scalability.
The RAN currently represents the telecom operators' largest resource allocation (including CapEx and OpEx). With ordinary radio base stations often operating at between 25% and 50% capacity, it also needs to be more utilized.
Main Benefits of O-RAN:
- Openness
- Interoperability
- Reduces vendor lock-in
- Allows for “best of breed” solutions
- Fosters greater innovation
O-RAN Architecture

Fig 1: O-RAN Architecture
O-RAN architecture enables disaggregation of RAN components, thereby enabling them to run on a cloud (on-prem, edge, or public clouds). Each component can come from a different vendor but they will interoperate as the interfaces are standard. Below is a high-level explanation of the main components:
- Service Management & Orchestrator (SMO)
- Near-RT RIC
- O-RU (O-RAN Radio Unit)
- O-DU (O-RAN Distributed Unit)
- O-CU (O-RAN Centralized Unit)
- O-Cloud (Open Cloud)
Role of O-RAN SMO
- O-CU, O-DU, and O-RU RAN functions are managed and orchestrated (Day-0/Day-N) by O-RAN SMO.
- It carries out FCAPS procedures in support of RAN tasks.
- The functionality of O-RAN SMO is a logical fit for AMCOP, which carries out:
- Orchestration (Day-0)
- (Day-N) LCM
- Service Assurance, or automation using Open Loop and Closed Loop
- AMCOP is a fully functional O-RAN SMO as well as capable of orchestrating other Network Functions.
RAN-in-the-Cloud
"RAN-in-the-Cloud" refers to the concept of deploying Radio Access Network (RAN) components in a cloud-based environment. Here the RAN functions, such as baseband processing and control functions, are virtualized and run on cloud infrastructure instead of dedicated hardware. This virtualization allows for more flexibility, scalability, and cost-efficiency in deploying and managing RAN networks. Instead of deploying and maintaining physical equipment at each cell site, RAN-in-the-Cloud enables centralized control and virtualized RAN functions that can serve multiple cell sites.
O-RAN-based implementations must use an end-to-end cloud architecture and be deployed in a genuine data center cloud or edge environment in order to be more valuable than proprietary RAN. RAN-in-the-Cloud is a 5G radio access network running as a containerized solution in a multi-tenant cloud architecture alongside other applications. These can be dynamically assigned in an E2E stack to improve utilization, lower CapEx and OpEx for telecom carriers, and enable the monetization of innovative edge services and applications like AI.
During periods of underutilization in a RAN-in-the-Cloud architecture, the cloud can run additional workloads. Power usage and effective CAPEX will both be greatly decreased. With the ability to support 4T4R, 32T32R, 64T64R, or TDD/FDD on the same infrastructure, the RAN will become versatile and programmable. Additionally, MNOs can employ a GPU-accelerated infrastructure for other services like Edge AI, video applications, CDN, and more when the RAN isn't being used to its full potential.
RAN-in-the-Cloud POC
This Proof of Concept (POC) was commissioned by Aarna.ml along with its partners Nvidia and Radisys. The left-hand side reflects a peak hour scenario, where there is an SMO, a CU and DU, and two servers. The Radio Units are connected through a switch. There is a 5G Core running with the SMO. During the off-peak hours (as shown by the right-hand side), when the utilization reduces, the vendor-neutral, intelligent SMO monitors the metrics of the underlying network, and based on certain predefined policies it can migrate some of the functions dynamically. For example, the DU2 and CU2 are utilizing 50% of the server capacity, then SMO migrates the DU to the first server and CU1 serves both the DUs. Since Server 2 gets freed up and thus it can be used for other applications (for example AI Edge App).
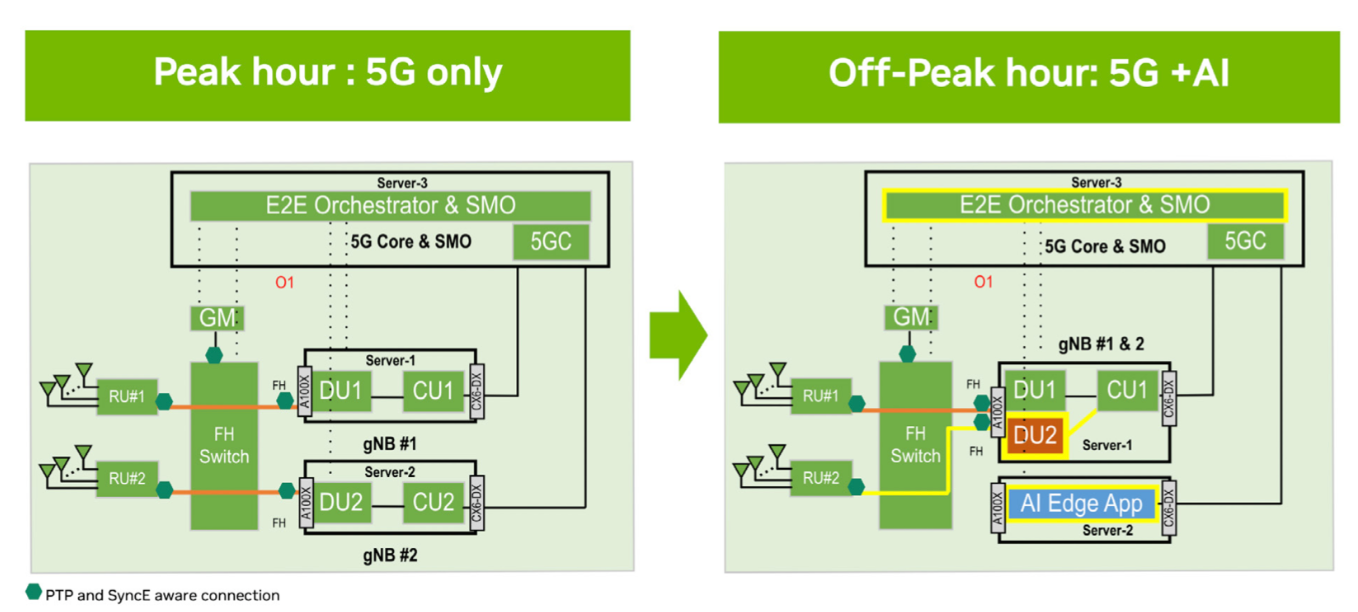
Fig 2: Enabling Multi-tenancy with Dynamic Orchestration of RAN Workload
AI/ML is a Game Changer
Artificial intelligence is revolutionizing every industry. Not only can a COTS platform with GPU accelerators speed up 5G RAN, but also can be used for AI/ML edge applications. This offers a simple method for speeding 5G RAN connection and AI applications using the same GPU resources. Network Operators running AI/ML applications with no additional resource cost, and using the output from the AI/ML models for critical business decisions will have a competitive advantage.
Role of AI in O-RAN
O-RAN Architecture supports interfaces for running applications through standard interfaces.
These applications can be developed by multiple vendors, thus encouraging a vendor-agnostic ecosystem. For example, rApps/xApps are developed by various vendors for RAN optimization.
Additionally, the RAN data or network data may be fed into a Data Lake and connected to an MLOps Engine for further data analysis. An additional function of AI and ML is what we discussed above – improve cloud (running RAN) resource utilization by using analytics and run cutting-edge AI applications using the same resources.
Requirements for AI/ML in O-RAN
- General purpose compute with a cloud layer such as Kubernetes
- General purpose acceleration, for example NVidia GPU, can be used by non-O-RAN workloads such as AI/ML, video services, CDNs, Edge IOT, and more
- Software defined xHaul and networking
- Vendor neutral SMO (Service Management and Orchestration) that can perform the dynamic switching of workloads from RAN→non-RAN→RAN
- The SMO also needs intelligence to understand how the utilization of the wireless network varies over time.
Sustainability is no Longer a Luxury
Reducing carbon emissions in network deployment is an important consideration for mitigating the impact of information and communication technology (ICT) infrastructure on the environment. Here are some strategies and technologies that can contribute to carbon reduction in network deployment:
- Opting for energy-efficient hardware
- Enabling multiple virtual network functions or services to run on a single physical server
- Designing and operating data centers with efficient cooling systems, optimized airflow management, energy-efficient servers, and use renewable energy sources to power data centers
- Transitioning to renewable energy sources such as solar, wind, or hydropower for powering network infrastructure
- Implementing energy management systems and monitoring tools to track and optimize energy consumption
- Using fiber-optic networks as they use less power to transmit data over longer distances
- Implementing energy-efficient protocols, such as Energy Efficient Ethernet (EEE)
- Optimizing network routing and traffic management to reduces unnecessary data transmission
- Encouraging infrastructure sharing and collaboration among network operators
- Properly manainge lifecycle of network equipment
Here, the SMO can have a dramatic impact on the O-RAN Capital Expenditure (CAPEX).
O-RAN implementations lend themselves to sustainability and energy efficiency by using:
- Standard off-the-shelf servers rather than specialized hardware logic
- Optimal resource utilization by leveraging them to run other tasks
- Software/Virtualization of RAN components reduces power consumption
- On-demand scaling is made simpler by container and VM workloads
For more details check the recording of the webinar. Subscribe to our YouTube Channel for more informative videos on O-RAN and its advantages. Get the RAN-in-the-Cloud Solution Brief.